DiffuScene: Scene Graph Denoising Diffusion Probabilistic Model for Generative Indoor Scene Synthesis
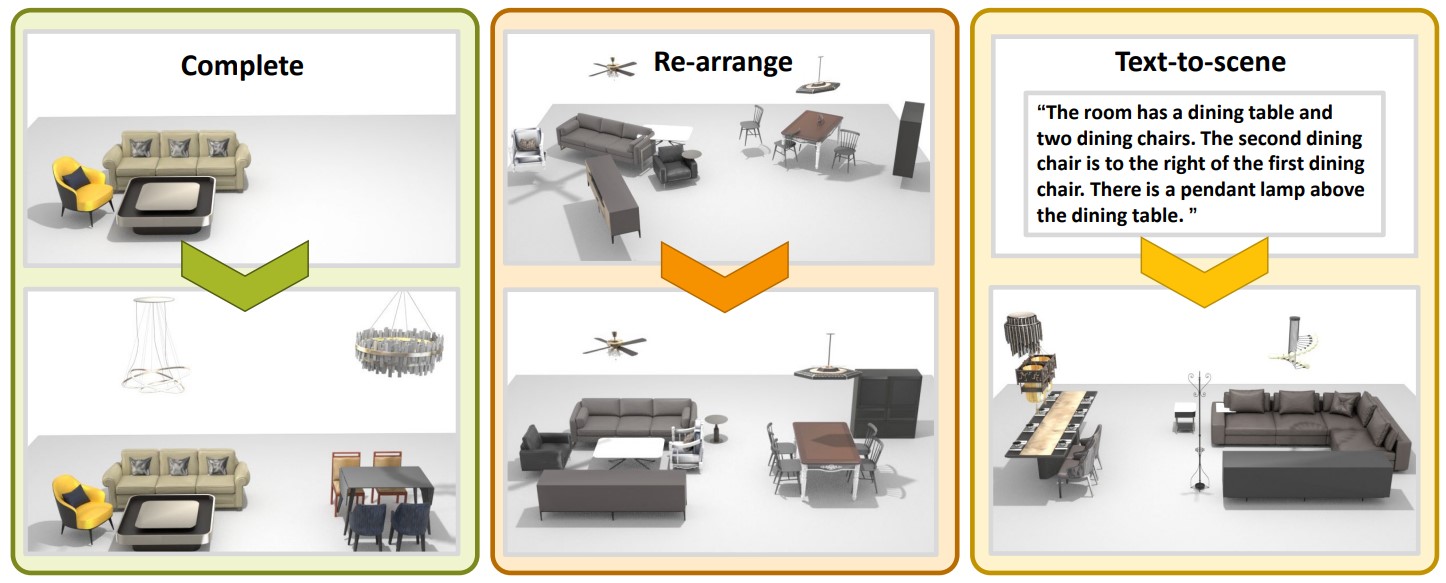
We present DiffuScene for indoor 3D scene synthesis based on a novel scene graph denoising diffusion probabilistic model, which generates 3D instance properties stored in a fully-connected scene graph and then retrieves the most similar object geometry for each graph node i.e. object instance which is characterized as a concatenation of different attributes, including location, size, orientation, semantic, and geometry features. Based on this scene graph, we designed a diffusion model to determine the placements and types of 3D instances. Our method can facilitate many downstream applications, including scene completion, scene arrangement, and text-conditioned scene synthesis. Experiments on the 3D-FRONT dataset show that our method can synthesize more physically plausible and diverse indoor scenes than state-of-the-art methods. Extensive ablation studies verify the effectiveness of our design choice in scene diffusion models.
[Paper] [Video] [Bibtex]